How to Train Your [Dragon] Embodied Agent (with AI Habitat)
ECCV 2020 Tutorial
Sunday, 23rd Augst
Online
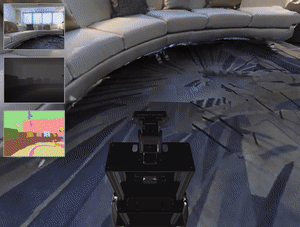
Overview
There has been a recent shift in the computer vision community from tasks focusing on internet images to active settings involving embodied agents that perceive and act within 3D environments. Practical deployment of AI agents in the real world requires study of active perception and coupling of perception with control as in embodied agents.
In this tutorial, we will demonstrate how to utilize the Habitat platform to train embodied agents to perform a variety of tasks in complex and photo-realistic environments.
Tutorial Content
Introduction to AI Habitat | Video | |
Navigation in Habitat | Video | |
Habitat-Sim Basics for Navigation | Video | Colab |
Habitat-Lab for Navigation | Video | Colab |
Habitat-Challenge | Video | Colab |
Habitat-Sim for Interaction | Video | Colab |
Habitat-Sim Advanced Topics | Video | Colab |
Habitat-Lab for Interaction | Video | Colab |
Faster RL Training: Profiling and Optimization | Video | Colab |
Welcome and introduction In this section, we will first cover the scientific motivation for embodied AI. We will cover the design philosophy and architecture of AI Habitat, to help potential users and researchers understand how AI Habitat views training virtual robots in simulated environments.
Task: Navigation in Habitat In this session we discuss, at a high level, training agents to perform navigation tasks (i.e. ‘Go to the bedroom’) in AI Habitat. Specifically, we discuss the task of PointGoal Navigation (i.e. go 5 meters forward and 2 meters to the left) and explain the embodiment of the agent, the deep neural network used to control it, how to frame the task as a reinforcement learning problem, and where Habitat-Sim and Habitat-Lab are used to train this agent with deep reinforcement learning.
Habitat-Sim Basics for Navigation Habitat-sim is a highly efficient, photorealistic 3D simulator for embodied agents operating in the virtual environments. This tutorial helps you to learn our simulator for navigation through a series of coding examples. It demonstrates how to load a scene, set and configure an agent, as well as its sensors (e.g., color, semantic, and depth sensors), instruct the agent to navigate and obtain the observations. It also introduces the NavMesh, an efficient mechanism to handle the collision constraints during the navigation. It teaches you how to load a NavMesh for the scene, recompute it at runtime, and let the agent take actions within the NavMesh.
Habitat-Lab for Navigation In this section we discuss how Habitat-Lab is used in defining embodied AI navigation tasks, evaluating agents, and training agents.
Habitat-Challenge In this section, we provide an overview of the Habitat Challenge – an AI challenge where participants upload agents to be evaluated in unseen environments.
Habitat-Sim for Interaction Habitat-sim is interactive! Users can now add new objects to a static background scene, set object states, run dynamics simulation, and more with ease through the Simulator API. This use-case driven tutorial covers interactivity in Habitat-sim. Topics such as adding objects to a scene, kinematic object manipulation, dynamics simulation, collision checking, and configurable navigation constraints will be covered. We will demonstrate a number of example applications, including: generating data for physical reasoning tasks, adding objects to the scene via rejection sampling on the NavMesh, and an embodied object fetch task.
Habitat-Sim Advanced Topics Expanding on the Habitat-Sim Basics and Interaction tutorials, this tutorial will cover some advanced topics for making the most of the Habitat-sim API. In addition to a detailed discussion of the object construction via attributes templates and the template libraries, we will demonstrate: tracking objects with a motion following camera, retrieving and displaying 2D projections of 3D points, and programmatically configuring semantic ids for objects and scene nodes.
Habitat-Lab for Interaction In this tutorial, we will look at how to setup an interaction task in Habitat Lab. We’ll cover key components using the task of furniture rearrangement as an example. We will walk through how to create, store and load objects into the simulator; define new action spaces such as a magic pointer action for grabbing / releasing objects; extend the observation space to include information about the objects and create reward functions for training RL agents. Finally we will train an RL agent to solve a single episode of this task. After going through this tutorial, one should be able to utilize all the interactive features of the Habitat Simulator and expose them through Habitat Lab in the form of observations, new action spaces, measurements and reward.
Faster RL Training: Profiling and Optimization Why focus on performance optimization for RL training? The goal is higher framerates. A higher framerate lets us scale our training to more steps, and it lets us iterate faster on our day-to-day experiments. In this short, accessible tutorial, we’ll profile and optimize an example program in real time in a Colab notebook. We’ll use one of Habitat’s PointGoal navigation baselines as our example program, but the lessons here can be applied to any RL training. We’ll walk through the process of capturing a profile, identifying candidates for optimizations, making improvements to our code, and evaluating speedup. We’ll try some profiling tools including py-spy, speedscope, and Nsight Systems. We’ll use a multithreaded trace to identify GPU-bound scenarios and opportunities for better parallelism.
Organizers
